Data is central to improving manufacturing processes. This is because in order to improve a process, manufacturing managers first need to understand the current state of the process in quantitative terms e.g. processing time, cycle time, units produced per hour. Once the current state is well defined, improvement plans can then be initiated and the outcomes measured against the current state to indicate if the improvement was realized.
With the rise of cloud computing it has become easier for manufacturers to store and process large amounts of production data without the need to manage a complex IT infrastructure. Cloud computing allows manufacturers to pay only for cloud services they use, helping them lower their operating costs, run their infrastructure more efficiently, and scale as their business needs change. However, a pure cloud computing system poses significant challenges in a production environment.
A far superior approach is to use a hybrid edge-cloud architecture for the processing and storage of data. This hybrid architecture combines the best of both worlds while minimizing the problems associated with each architecture as a stand-alone option.
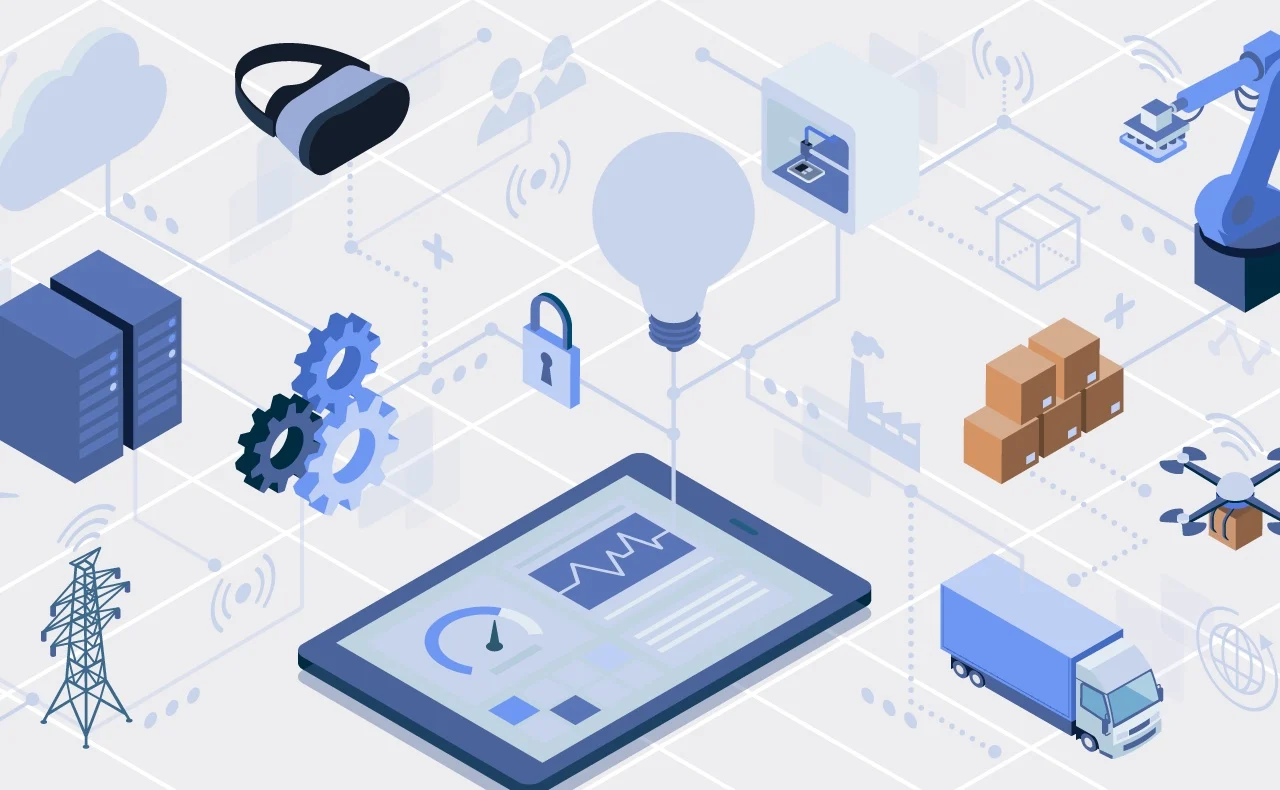